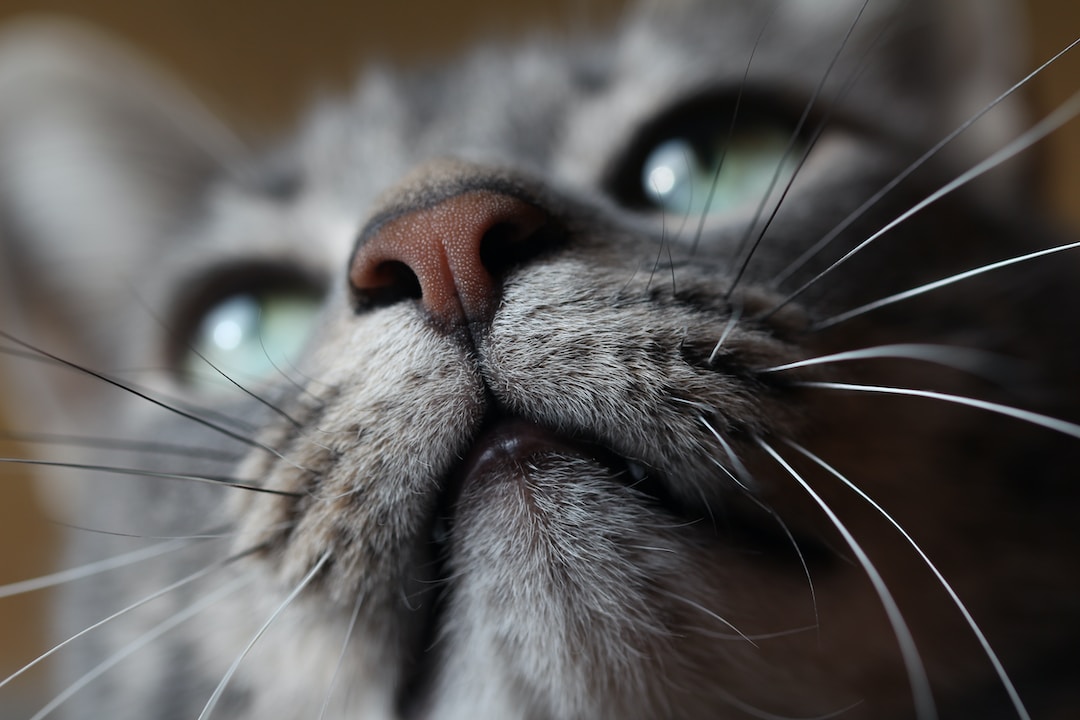
Visualizing data can be a daunting task if you’re new to the realm of data science. Nonetheless, it remains a critical aspect of data analysis. One tool often used in this realm is the box plot. Intricate yet simple, a box plot is a graphical representation that provides a snapshot of a set of data. In this article, we will uncover the principles, construction, utilization, and complexities associated with box plots.
Understanding the Fundamentals of Box Plots (Whisker Plots)
A box plot, also known as a whisker plot, is a type of chart that displays the five-number summary of a set of data—minimum, first quartile, median, third quartile, and maximum. It provides a comprehensive view of the data distribution and its dispersion.
Getting a good grip on box plots means understanding that they are suited for comparing distributions across groups. They graphically depict groups of numerical data through their quartiles, providing an easy way to visualize trends and compare different data sets.
To effectively use box plots in data interpretation, one must understand the underlying principles guiding its construction. Knowledge of these principles aids in accurate data representation and interpretation.
The Components of a Box Plot: A Detailed Analysis
A box plot consists of various components—the box, the lines, otherwise known as the whiskers, and the dots. The box itself represents the interquartile range (IQR), which is a measure of statistical dispersion.
The median is the line that divides the box into two parts. Above and below the box, you have the upper and lower whiskers, which stretch to the highest and lowest data points within 1.5 times the IQR from the box.
This technique minimizes the impact of outliers—extreme values that can distort the overall picture of the data. The dots that lie beyond the whiskers are considered outliers.
Knowing what each component represents is fundamental to the science of box plots, allowing for precise data visualization and comparative analysis.
The Process of Creating Box Plots: Step-by-Step Approach
Creating a box plot involves various stages and can be done either manually or with the help of computational tools. If done manually, the first step is data collection, followed by data categorization into quartiles.
Next is the calculation of the five-number summary, followed by the construction of a scale reflecting the maximum, minimum, and median. Finally, the box is drawn along with the whiskers to illustrate the data.
In the digital age, however, numerous programs and software can aid in the construction of box plots. These computational tools simplify the process by automatically calculating the five-number summary and creating the corresponding visual representation.
The convenience and accuracy that these programs provide make them a popular choice among data analysts. Thus, despite the underlying mathematical processes involved, creating box plots has become more feasible and less arduous.
Overcoming Complexities: Deciphering Outliers in Box Plots
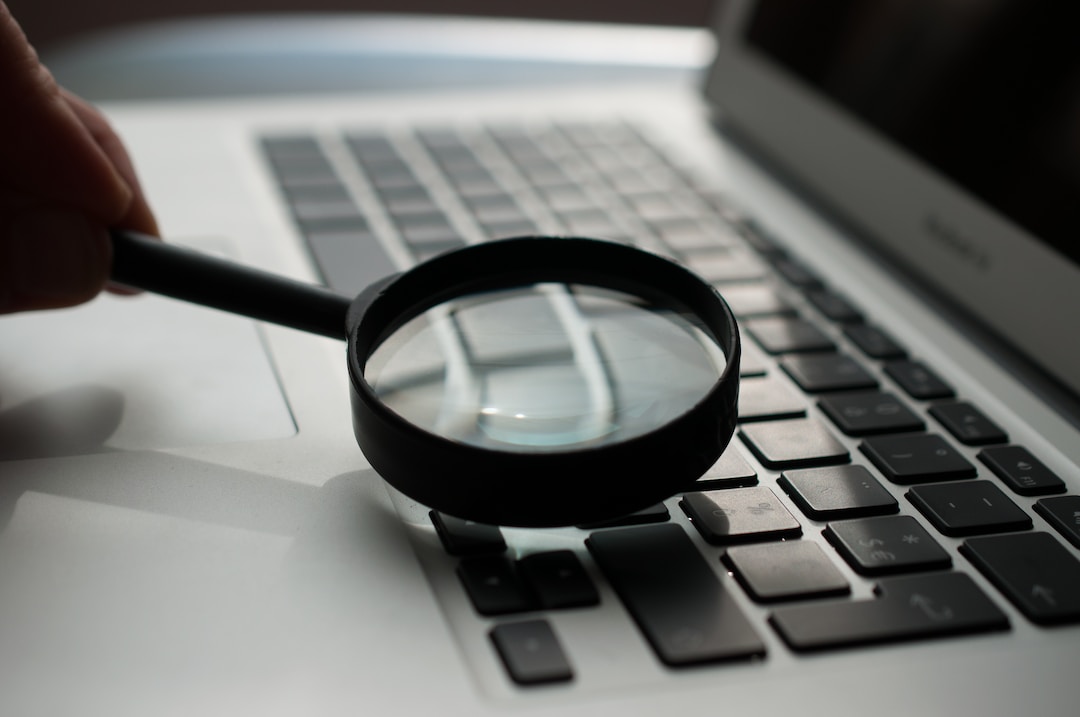
One of the complexities associated with box plots involves the identification and interpretation of outliers. Since outliers can heavily impact the interpretation of data, how they are represented and understood in a box plot is vital.
Box plots handle outliers by indicating them as points outside the whiskers. By simplifying detection, it allows for their effects on the data to be minimized or accounted for.
While the interpretation of outliers should be handled with caution, as they sometimes could be indications of data errors, they could also represent a true reflection of the phenomenon under investigation.
Understanding this complexity and learning how to decipher outliers in box plots is a skill that enhances the accuracy and reliability of data interpretations.
Altogether, understanding, constructing, and interpreting box plots can greatly enhance the way data is perceived and analyzed. Knowing what a box plot is and its components, how to create one, their usage, and how to handle outliers are key skills that every data analyst should master. It not only simplifies data visualization but also makes complex data interpretable and actionable.